3 Ways Machine Learning Can Enhance Insurance Underwriting
The day-to-day work of an Underwriter ranges from research, to data entry, to pricing a risk, to ultimately negotiating that premium value with an agent. At the core, they need to accurately gauge risk, on a case by case basis. But their job doesn’t stop there. Even if we were to codify all the significant risk factors (as actuarial tables do), this doesn’t translate directly to how much the insurance firm ultimately charges for a given premium. Underwriters need to create an offer that they can justify to their customers, and keep an eye on the prevailing market dynamics.
Underwriters navigate a sea of data, software, and file formats to reach a premium for each policy. It’s challenging work, with many uncertainties. In our experience, Machine Learning can be used to enhance the insurance underwriting process in a number of ways.
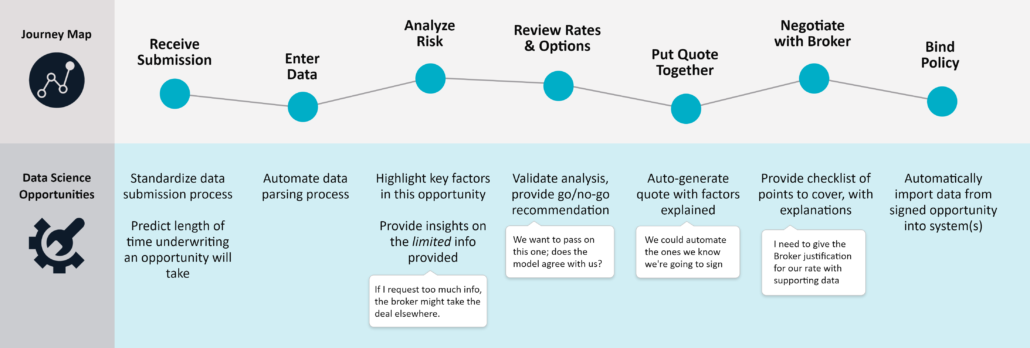
Let’s walk through the three most valuable applications for Machine Learning in Insurance:
- Analyzing an individual’s claim risk
- Predicting the severity of a claim or incident
- Generating a quote
Note: We will use medical liability insurance to provide context and examples, but these tasks and the application of machine learning would be similar/applicable across medical, P&C or other types of insurance.
Underwriter Task 1: Analyze an individual’s claim risk
When an Underwriter adds a new policy to their book of business, they are taking on risk. What is the likelihood that there will be a claim on this policy in the future? A perfect world for an Underwriter would be to know with certainty that someone they are insuring will have a claim in the near future. Risk prediction may never be reach this state, as reality contains many unquantifiable factors and random events. The more we know about who we are insuring and what they are doing, the better we can gauge the amount of risk we are adding to the book.
During this process, Underwriters assess risk based on the individual’s history, as well as other factors that are deemed important predictors. Underwriters draw on their own experience and the experience of their teammates to predict if an insured will have a claim. Bias is inadvertently involved, since every Underwriter has a different perspective.
Machine Learning Opportunity: Predict the likelihood of a future claim
A long, ever expanding list of factors may influence the risk of an individual. Some factors may be proxies for less quantifiable information, while others may simply be things that an Underwriter never thought to consider.
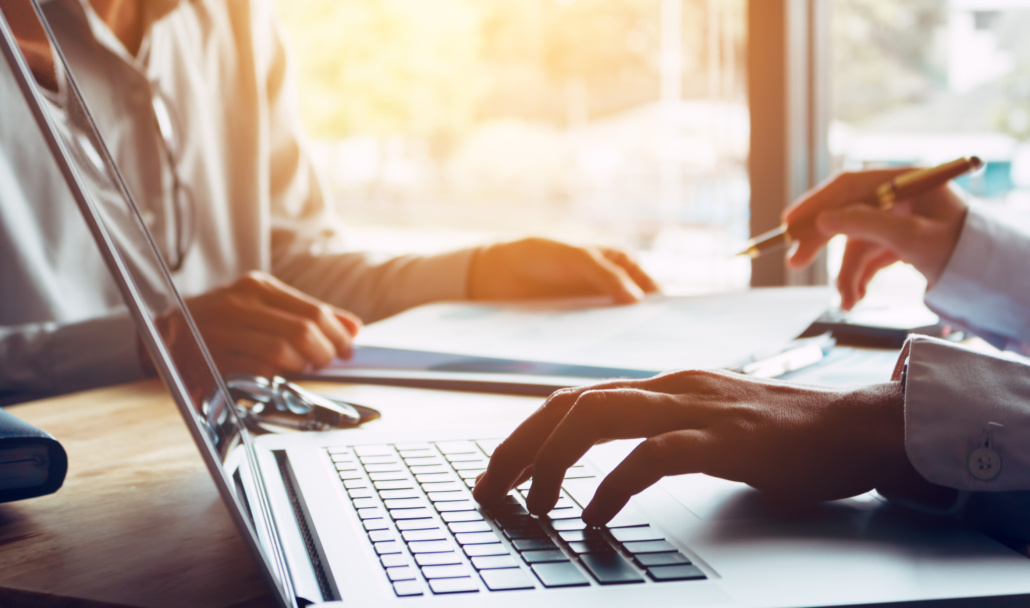
By gathering all company data and including third party data sources, a machine learning model can garner a more comprehensive view of the insured. A data science model can help better classify and determine quantifiable risk factors, and help the Underwriter grapple with this complexity. Through a classification model, the model can see if an individual has similar characteristics to others in the population with claims filed. A match with this pattern would enable the Underwriter to increase the premium.
Incorporating intelligent machine learning models in the underwriting process not only helps train Underwriters to view risk factors more confidently, but helps standardized the risk scoring process across a company. Training can only go so far to help keep risk scoring consistent, so machine learning can help prevent insurance companies from taking on too much risk.
Underwriter Task 2: Predict the outcome of a claim or incident
There is a broad range in severity and expenditures for different claims. In the case of medical liability insurance, doctors who are part of a group policy may be listed on a claim even if they were not personally involved in the incident. Frivolous claims might only incur minor legal expenses, but a legitimate claim might result in a massive payout. This complexity makes it challenging to understand the severity of a claim filed against an individual, and has a massive impact on the risk that an insurance firm undertakes.
Machine Learning Opportunity: Predict outcomes for open claims
Understanding how to differentiate between the large spread of claim severity can help more accurately predict how much an incident will cost the insurance firm. One factor to consider is that certain specialties may have lawsuits that consistently go to trial, while others may not. Some regions may have a very high frivolous claim rate, but these suits typically cost insurance companies nothing more than small legal fees. Some states have tort reform laws that make it very easy to settle at a low expense. Understanding the severity of a claim, and not just the chance of occurrence, directly contributes toward predicting the exposure of a firm.
Underwriter Task 3: Generate a quote
If you were to watch an Underwriter work, you would notice that they don’t spend equal amounts of time on every quote they write. Sometimes the quote they generate requires a fine-grain analysis and explanation, such as when a Physician provides an innovative service, or works in an unusual context. Other times, an opportunity might be more “by the book,” and the high-touch underwriting process can feel excessive. This can be especially true in the renewal process.
Year to year, some renewals may not contain any changes in application information. The doctor may see roughly the same number of patients, maintain the same specialty in practice, and has had no claims filed against him or her. An Underwriter still has to review all of this information to confirm it is true, which is a time consuming process that leads to the same premium amount.
Machine Learning Opportunity: Auto-generate risk quote
A machine learning model can be utilized to translate these risk factors into a suggested premium based on all of the historical data included in the model. In the case of renewals, a machine learning model can determine is there are any changes to the most important risk factors, which can then translate directly into an auto-generated quote that will be the same as the previous year.
This helps insurance firms standardize the rate they charge across the firm, ultimately removing any bias that may leak into a premium calculation. It also provides a good gut-check to Underwriters so they know that their assumptions led them to roughly the right premium amount. And finally, it would allow Underwriters to save time on these remedial applications, giving them more time to spend properly analyzing new or unique prospects.
The insurance industry will likely never get to a state of zero uncertainty in underwriting. But machine learning can help us to get a bit closer. By pairing Data Science with the deep well of an Underwriter’s experience and expertise, we can increase Underwriter efficiency and confidence, and promote standardization across the firm.
Most importantly, quality of prediction is a strategic advantage. Creating better methods to reduce and manage risk is essential, and can make the difference between a profitable and unprofitable insurance business.
If you enjoyed this article and want to read more written by Joe Sniezek, view his collection.
About Ironside
Ironside was founded in 1999 as an enterprise data and analytics solution provider and system integrator. Our clients hire us to acquire, enrich and measure their data so they can make smarter, better decisions about their business. No matter your industry or specific business challenges, Ironside has the experience, perspective and agility to help transform your analytic environment.